
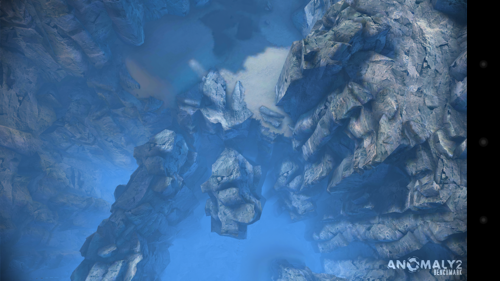
However, in the real world of manufacturing or medicine, the distinction between normal and abnormal images is finer and subtler than these object class distinctions. Usually set one category in CIFAR-10 dataset as the normal class and the rest as anomalies.

Previous studies focus on the unsupervised anomaly detection task and success in abstracting semantically rich representation for isolating defect images, nonetheless, they lack the ability to explore the fine-grained anomalies. To this end, unsupervised anomaly detection and localization task has been proposed, which aims to develop computational models and techniques that detect and localize anomalies without using any anomaly data during training. Limited by the data scarcity problem of anomaly data and theĮxtremely uneven distribution among normal and abnormal classes, supervised learning based methods are difficult to apply, especially deep learning methods. Image anomaly detection and localization is an important computer vision task that deals with detecting and localization unexpected or abnormal patterns in digital images, which has been widely used in manufacturing and healthcare On this new dataset, and reports the corresponding results with analysis. Paper also conducts experiments using the existing state-of-the-art 2D methods Moreover, considering the proposal of the new MVTec 3D-AD dataset, this
Anomaly 2 benchmark full#
Meanwhile, analysis of the MVTec AD dataset are also given,Įspecially the label ambiguity that affects the model fails to achieve full Tasks, and adds a comparison of inference efficiency previously ignored by theĬommunity. In terms of the performance in unsupervised anomaly detection and localization This paper extensively compares 13 papers It is the time to conduct a comprehensive comparison of existing New research methods that are constantly being proposed push its precision to From the time the MVTec AD dataset was proposed to the present, Unsupervised anomaly detection and localization, as of one the most practicalĪnd challenging problems in computer vision, has received great attention in
